Category Associate
Exam duration 130 minutes
Exam format 65 questions
Cost USD. Visit Exam pricing for additional cost information, including foreign exchange rates
Intended candidate Individuals with at least 1 year of experience using Amazon SageMaker and other ML engineering AWS services
Candidate role examples backend software developer, DevOps engineer, data engineer, MLOps engineer, and data scientist
Languages offered English, Japanese, Korean, and Simplified Chinese
Level: Associate
Format: 65 questions (multiple-choice, multiple-response, matching, ordering, and case studies)
Duration: 130 minutes
Passing Score: Scaled score of 720 out of 1000
Intended Audience
This certification is ideal for professionals with at least one year of experience in roles such as:
* Backend Software Developer
* DevOps Engineer
* Data Engineer
* MLOps Engineer
* Data Scientist([Amazon Web Services, Inc.
Candidates should have hands-on experience with Amazon SageMaker and other AWS ML services.
Examkingdom Amazon Associate MLA-C01 Exam pdf
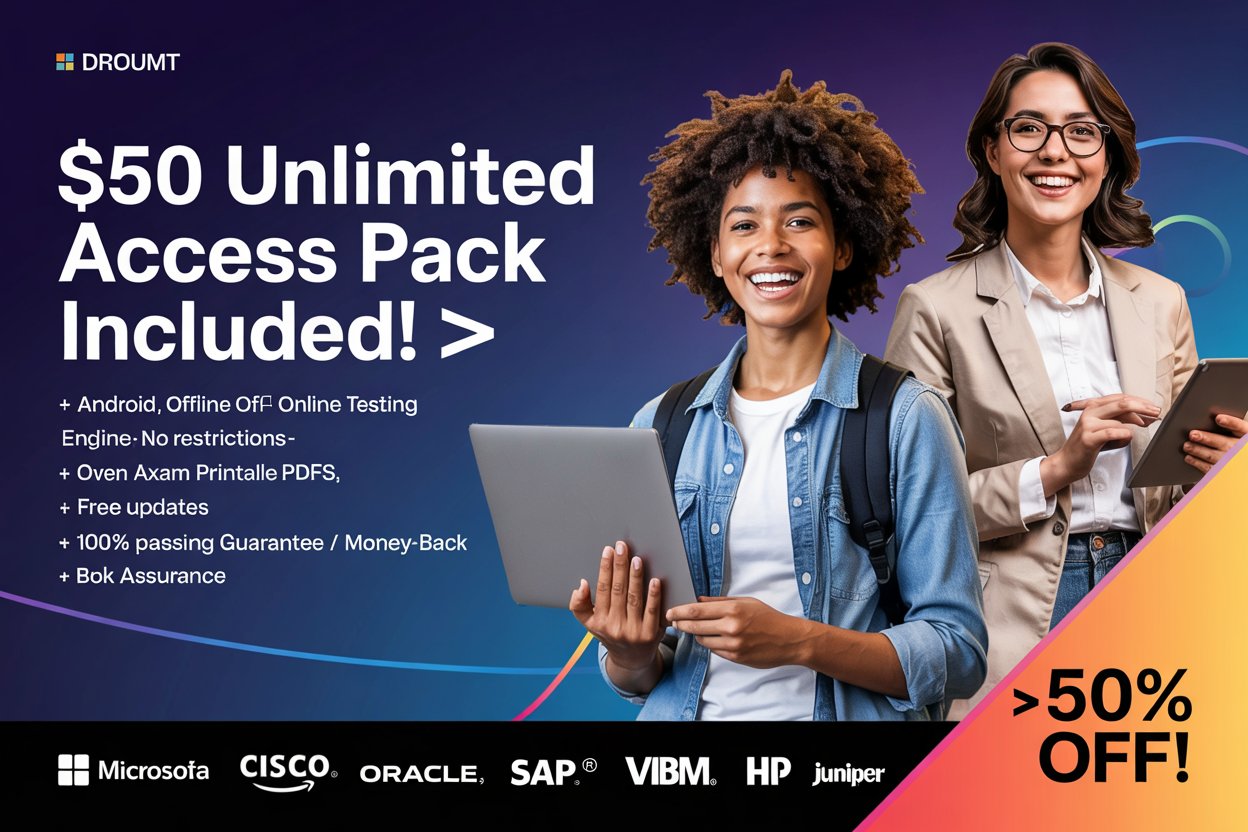
Best Amazon Associate MLA-C01 Downloads, Amazon Associate MLA-C01 Dumps at Certkingdom.com
Exam Domains
The exam covers four key domains:
1. Data Preparation for Machine Learning
* Ingesting, transforming, and validating data for ML modeling.
2. ML Model Development
* Selecting modeling approaches, training models, tuning hyperparameters, and managing model versions.
3. Deployment and Orchestration of ML Workloads
* Choosing deployment infrastructure, provisioning compute resources, and configuring auto-scaling.
4. ML Solution Monitoring, Maintenance, and Security
* Setting up CI/CD pipelines, monitoring models and infrastructure, and ensuring security compliance.
Preparation Resources
To prepare effectively for the MLA-C01 exam, consider the following resources:
AWS Exam Guide: Provides detailed information about the exam structure and content.
AWS Skill Builder: Offers a structured exam prep plan with practice questions.
AWS Certified Machine Learning Engineer – Associate validates technical ability in implementing ML workloads in production and operationalizing them. Boost your career profile and credibility, and position yourself for in-demand machine learning job roles.
Prepare for the exam
Go from start to certified. Follow our Exam Prep Plan on AWS Skill Builder, our online learning center, so you can approach exam day with confidence.
1 Get to know the exam with exam-style questions
Follow the 4-step plan.
Review the exam guide.
Take the AWS Certification Official Practice Question Set to understand exam-style questions.
2 Refresh your AWS knowledge and skills
Enroll in digital courses where you need to fill gaps in knowledge and skills, practice with AWS Builder Labs, AWS Cloud Quest, and AWS Jam.
3 Review and practice for your exam
Review the scope of the exam. Explore each exam domain’s topics and how they align to AWS services. Reinforce your knowledge and identify learning gaps with exam-style questions and flashcards. Follow instructors as they walk through exam-style questions and provide test-taking strategies. Continue practicing with AWS Builder Labs and/or AWS SimuLearn.
4 Assess your exam readiness
Take the AWS Certification Official Pretest.
Key FAQs to help you get started
Who should earn AWS Certified Machine Learning Engineer – Associate?
The ideal candidate for this exam has at least 1 year of experience in machine learning engineering or a related field and 1 year of hands-on experience with AWS services. Professionals who do not have prior machine learning experience can take the training available in the Exam Prep Plans and get started building their knowledge and skills.
How will the AWS Certified Machine Learning Engineer – Associate help my career?
Per the World Economic Forum Future of Jobs Report 2023, demand for AI and Machine Learning Specialists is expected to grow by 40%. However, 70% of North American IT leaders say they have the greatest difficulty filling AI/ML specialist roles. This certification can position you for in-demand machine learning jobs in AWS Cloud.
How is AWS Certified Machine Learning Engineer – Associate different from AWS Certified Machine Learning – Specialty?
AWS Certified Machine Learning Engineer – Associate is a role-based certification designed for ML engineers and MLOps engineers with at least one year of experience in AI/ML.
AWS Machine Learning – Specialty is a specialty certification covering topics across data engineering, data analysis, modeling, and ML implementation and ops. It is more suitable for individuals with 2 or more years of experience developing, architecting, and running ML workloads on AWS.
What certification(s) should I earn next after AWS Certified Machine Learning Engineer – Associate?
For professionals looking to dive deeper into machine learning, we recommend AWS Certified Machine Learning – Specialty.
How long is this certification valid for?
This certification is valid for 3 years. Before your certification expires, you can recertify by passing the latest version of this exam. Learn more about recertification options for AWS Certifications.
Sample Question and Answers
QUESTION 1
Case Study
A company is building a web-based AI application by using Amazon SageMaker.
The application will provide the following capabilities and features: ML experimentation, training, a
central model registry, model deployment, and model monitoring.
The application must ensure secure and isolated use of training data during the ML lifecycle.
The training data is stored in Amazon S3.
The company needs to use the central model registry to manage different versions of models in the application.
Which action will meet this requirement with the LEAST operational overhead?
A. Create a separate Amazon Elastic Container Registry (Amazon ECR) repository for each model.
B. Use Amazon Elastic Container Registry (Amazon ECR) and unique tags for each model version.
C. Use the SageMaker Model Registry and model groups to catalogthe models.
D. Use the SageMaker Model Registry and unique tags for each model version.
Answer: C
QUESTION 2
Case Study
A company is building a web-based AI application by using Amazon SageMaker.
The application will provide the following capabilities and features: ML experimentation, training, a central model registry, model deployment, and model monitoring.
The application must ensure secure and isolated use of training data during the ML lifecycle.
The training data is stored in Amazon S3.
The company is experimenting with consecutive training jobs.
How can the company MINIMIZE infrastructure startup times for these jobs?
A. Use Managed Spot Training.
B. Use SageMaker managed warm pools.
C. Use SageMaker Training Compiler.
D. Use the SageMaker distributed data parallelism (SMDDP) library.
Answer: B
QUESTION 3
Case Study
A company is building a web-based AI application by using Amazon SageMaker.
The application will provide the following capabilities and features: ML experimentation, training,
a central model registry, model deployment, and model monitoring.
The application must ensure secure and isolated use of training data during the ML lifecycle.
The training data is stored in Amazon S3.
The company must implement a manual approval-based workflow to ensure that only approved models can be deployed to production endpoints.
Which solution will meet this requirement?
A. Use SageMaker Experiments to facilitate the approval process during model registration.
B. Use SageMaker ML Lineage Tracking on the central model registry. Create tracking entities for the approval process.
C. Use SageMaker Model Monitor to evaluate the performance of the model and to manage the approval.
D. Use SageMaker Pipelines. When a model version is registered, use the AWS SDK to change the approval status to “Approved.”
Answer: D
QUESTION 4
Case Study
A company is building a web-based AI application by using Amazon SageMaker.
The application will provide the following capabilities and features: ML experimentation, training, a central model registry, model deployment, and model monitoring.
The application must ensure secure and isolated use of training data during the ML lifecycle.
The training data is stored in Amazon S3.
The company needs to run an on-demand workflow to monitor bias drift for models that are deployed to real-time endpoints from the application.
Which action will meet this requirement?
A. Configure the application to invoke an AWS Lambda function that runs a SageMaker Clarify job.
B. Invoke an AWS Lambda function to pull the sagemaker-model-monitor-analyzer built-in SageMaker image.
C. Use AWS Glue Data Quality to monitor bias.
D. Use SageMaker notebooks to compare the bias.
Answer: A
QUESTION 5
HOTSPOT
A company stores historical data in .csv files in Amazon S3. Only some of the rows and columns in the .csv files are populated.
The columns are not labeled. An ML engineer needs to prepare and store the data so that the company can use the data to train ML models.
Select and order the correct steps from the following list to perform this task. Each step should be
selected one time or not at all. (Select and order three.)
Create an Amazon SageMaker batch transform job for data cleaning and feature engineering.
Store the resulting data back in Amazon S3.
Use Amazon Athena to infer the schemas and available columns.
Use AWS Glue crawlers to infer the schemas and available columns.
Use AWS Glue DataBrew for data cleaning and feature engineering.
Answer:
Explanation:
Step 1: Use AWS Glue crawlers to infer the schemas and available columns.
Step 2: Use AWS Glue DataBrew for data cleaning and feature engineering.
Step 3: Store the resulting dat back in Amazon 89
Post your comments